In today's data-driven world, integrated statistics has become an essential tool for businesses, researchers, and analysts. It combines various statistical techniques and methodologies to provide a holistic approach to data analysis. Whether you're working in finance, healthcare, or technology, understanding integrated statistics can significantly enhance your decision-making process.
Integrated statistics is not just about crunching numbers; it's about deriving meaningful insights that drive innovation and growth. By leveraging advanced algorithms, machine learning, and data visualization tools, integrated statistics helps organizations unlock the full potential of their data assets. This article will delve into the core principles of integrated statistics, its applications, and how it can benefit your business.
As we navigate through this comprehensive guide, we will explore the key components of integrated statistics, its importance in modern analytics, and how it aligns with current industry trends. By the end of this article, you'll have a clear understanding of how integrated statistics can transform the way you approach data analysis.
Read also:Diva Flawless Nude
Table of Contents
- What is Integrated Statistics?
- Key Components of Integrated Statistics
- The Importance of Integrated Statistics
- Applications of Integrated Statistics
- Challenges in Implementing Integrated Statistics
- Tools and Technologies for Integrated Statistics
- Data Visualization in Integrated Statistics
- Current Industry Trends in Integrated Statistics
- Future Directions in Integrated Statistics
- Conclusion
What is Integrated Statistics?
Integrated statistics refers to the combination of diverse statistical methods and tools to analyze complex data sets comprehensively. Unlike traditional statistical approaches, which often focus on isolated data sets or single methodologies, integrated statistics takes a multidimensional approach. It involves merging data from multiple sources and applying advanced techniques such as predictive modeling, machine learning, and Bayesian analysis.
This approach allows analysts to gain deeper insights into data patterns and relationships, leading to more accurate predictions and better decision-making. By integrating various statistical techniques, organizations can address complex problems that would be challenging to solve with conventional methods.
For example, in the healthcare industry, integrated statistics can combine patient data from electronic health records, clinical trials, and wearable devices to predict disease progression and optimize treatment plans. This holistic approach not only improves patient outcomes but also enhances operational efficiency.
Key Components of Integrated Statistics
Data Integration
Data integration is one of the foundational components of integrated statistics. It involves consolidating data from different sources into a unified format, ensuring consistency and accuracy. This process often requires data cleaning, normalization, and transformation to make the data suitable for analysis.
Statistical Techniques
The use of advanced statistical techniques is another critical component. These include regression analysis, time series forecasting, clustering, and classification algorithms. Each technique serves a specific purpose, and their integration enables a more comprehensive analysis of data.
Machine Learning
Machine learning plays a significant role in integrated statistics, particularly in handling large and complex data sets. Algorithms such as neural networks, decision trees, and support vector machines can identify patterns and relationships that traditional methods might miss.
Read also:5starsstocks Com Passive Stocks
The Importance of Integrated Statistics
Integrated statistics is crucial for several reasons. First, it helps organizations make sense of the vast amounts of data generated daily. By combining multiple data sources and analytical techniques, businesses can uncover hidden insights that drive innovation and competitive advantage.
Second, integrated statistics enhances the accuracy and reliability of predictions. By leveraging diverse methodologies, analysts can validate their findings and ensure that their conclusions are robust and trustworthy.
Finally, integrated statistics supports better decision-making. Whether you're forecasting market trends, optimizing supply chains, or personalizing customer experiences, integrated statistics provides the tools and insights needed to succeed in today's fast-paced environment.
Applications of Integrated Statistics
Finance
In the financial sector, integrated statistics is used for risk assessment, portfolio optimization, and fraud detection. By analyzing historical data and market trends, financial analysts can predict potential risks and develop strategies to mitigate them.
Healthcare
As mentioned earlier, healthcare benefits significantly from integrated statistics. It enables personalized medicine, predictive diagnostics, and resource optimization. For instance, hospitals can use integrated statistics to forecast patient admissions and allocate resources more efficiently.
Retail
Retailers use integrated statistics to understand consumer behavior, optimize pricing strategies, and improve inventory management. By analyzing sales data, customer feedback, and market trends, retailers can enhance customer satisfaction and increase profitability.
Challenges in Implementing Integrated Statistics
While integrated statistics offers numerous benefits, its implementation comes with challenges. One major challenge is data quality. Inconsistent or incomplete data can lead to inaccurate results, undermining the effectiveness of integrated statistics.
Another challenge is the complexity of integrating multiple statistical techniques. Analysts need to possess a deep understanding of various methodologies and how they interact with each other. This requires specialized training and expertise.
Finally, there is the issue of scalability. As data volumes continue to grow, organizations must ensure that their integrated statistics systems can handle large-scale analyses without compromising performance.
Tools and Technologies for Integrated Statistics
Several tools and technologies support integrated statistics. These include:
- R and Python: Popular programming languages for statistical analysis and machine learning.
- SAS: A powerful software suite for advanced analytics, business intelligence, and data management.
- Tableau: A data visualization tool that helps analysts create interactive and shareable dashboards.
- Apache Spark: A distributed computing framework for processing large data sets in real-time.
These tools provide the necessary capabilities for data integration, analysis, and visualization, enabling organizations to harness the full potential of integrated statistics.
Data Visualization in Integrated Statistics
Data visualization is an integral part of integrated statistics. It transforms complex data into understandable visual representations, such as charts, graphs, and heatmaps. This makes it easier for stakeholders to interpret the results and make informed decisions.
Effective data visualization also enhances communication between analysts and non-technical audiences. By presenting data in a visually appealing and intuitive manner, organizations can bridge the gap between technical expertise and business strategy.
Current Industry Trends in Integrated Statistics
The field of integrated statistics is evolving rapidly, driven by advancements in technology and changing business needs. Some of the current trends include:
- Increased adoption of artificial intelligence and machine learning in statistical analysis.
- Growing emphasis on real-time data processing and analysis.
- Expansion of cloud-based analytics platforms for scalability and flexibility.
- Rise of open-source tools and libraries for statistical computing.
These trends reflect the growing demand for more sophisticated and accessible tools to support integrated statistics.
Future Directions in Integrated Statistics
The future of integrated statistics looks promising, with ongoing developments in technology and methodology. Some potential directions include:
- Integration of quantum computing for ultra-fast data analysis.
- Development of more intuitive and user-friendly interfaces for data visualization.
- Expansion into new domains such as environmental science and social media analytics.
- Enhanced focus on ethical considerations in data collection and analysis.
As technology continues to advance, integrated statistics will undoubtedly play an increasingly important role in shaping the future of data analysis.
Conclusion
In conclusion, integrated statistics represents a powerful approach to data analysis that combines diverse methodologies and tools to provide comprehensive insights. Its applications span across industries, offering solutions to complex problems and driving innovation. While challenges exist in its implementation, the benefits far outweigh the difficulties.
We encourage you to explore the possibilities of integrated statistics in your organization. Whether you're a seasoned analyst or a business leader, understanding this approach can help you unlock new opportunities and achieve greater success. Share your thoughts and experiences in the comments below, and don't forget to explore other articles on our site for more insights into the world of data analysis.
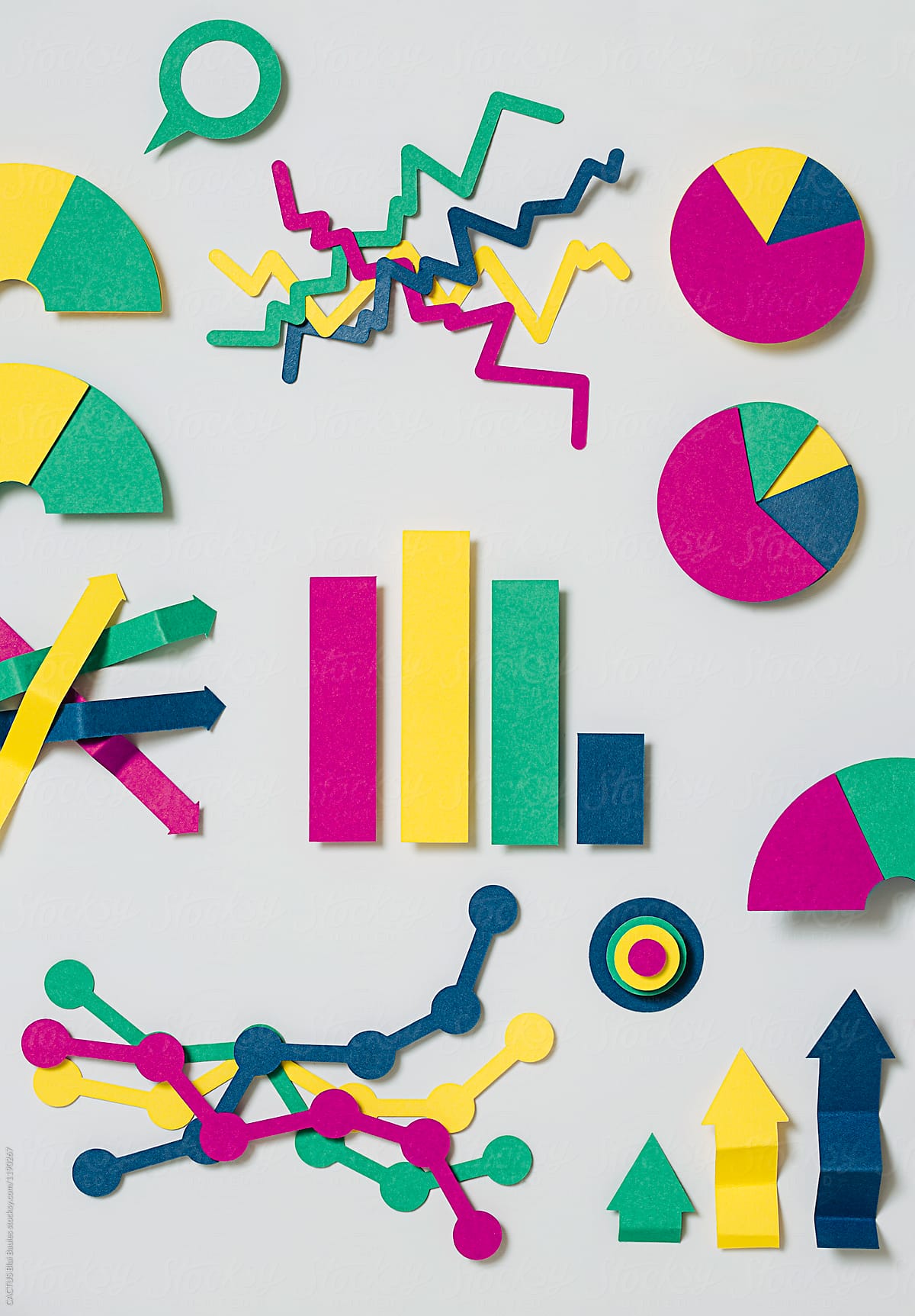