In the rapidly evolving world of artificial intelligence, multimodal Large Language Models (LLMs) have become a focal point for innovation. These advanced systems are designed to process and generate content across multiple modalities, such as text, images, audio, and video. However, despite their impressive capabilities, multimodal LLMs face significant challenges, particularly in their visual processing abilities. This article delves deep into the visual shortcomings of these models and explores why they matter.
As AI continues to integrate into everyday life, understanding the limitations of these systems becomes crucial. The visual shortcomings of multimodal LLMs can impact their effectiveness in applications ranging from healthcare to autonomous vehicles. By understanding these limitations, developers can work towards creating more robust and reliable models.
This article is structured to provide a comprehensive overview of the topic, including an exploration of the current state of multimodal LLMs, their visual processing challenges, and potential solutions. Whether you're a researcher, developer, or simply curious about AI, this article will provide valuable insights into the evolving landscape of multimodal AI systems.
Read also:Mydesi Org
Table of Contents
- Introduction to Multimodal LLMs
- The Role of Visual Data in AI
- Visual Shortcomings of Multimodal LLMs
- Impact on Real-World Applications
- Causes of Visual Shortcomings
- Current Research and Developments
- Potential Solutions
- Challenges Ahead
- Conclusion and Future Directions
- Call to Action
Introduction to Multimodal LLMs
Multimodal LLMs represent the next frontier in artificial intelligence. These models are designed to process information from multiple sources, such as text and images, simultaneously. By integrating these different forms of data, multimodal LLMs aim to replicate the way humans process information, providing a more holistic and context-aware understanding of the world.
However, while these models excel in processing textual data, their ability to handle visual information remains a challenge. This limitation affects their overall performance and reliability, particularly in applications where visual data plays a critical role.
The Role of Visual Data in AI
Visual data is a cornerstone of many AI applications. From medical imaging to autonomous driving, the ability to accurately interpret and analyze visual information is essential. Multimodal LLMs are expected to handle this data seamlessly, but their current capabilities often fall short of expectations.
Understanding the role of visual data in AI is crucial for identifying the gaps in current multimodal models and developing strategies to address these shortcomings.
Visual Shortcomings of Multimodal LLMs
Image Recognition Issues
One of the primary challenges faced by multimodal LLMs is image recognition. These models often struggle to accurately identify objects within images, leading to misinterpretations and errors. This issue is exacerbated by the complexity of real-world images, which can include occlusions, varying lighting conditions, and unusual perspectives.
Contextual Misunderstanding
Beyond simple recognition, multimodal LLMs often fail to understand the context in which visual data is presented. This can lead to misunderstandings and incorrect inferences, impacting the model's ability to provide accurate and meaningful outputs.
Read also:Camilla Araujo Naked
Impact on Real-World Applications
The visual shortcomings of multimodal LLMs have significant implications for real-world applications. In healthcare, for example, inaccurate image analysis can lead to misdiagnoses. In autonomous driving, misinterpretations of visual data can result in accidents. Understanding these impacts is essential for developing solutions that address these challenges.
Causes of Visual Shortcomings
The visual shortcomings of multimodal LLMs can be attributed to several factors. These include insufficient training data, limitations in model architecture, and the complexity of visual data itself. Addressing these causes requires a multifaceted approach that involves improvements in both data and model design.
Current Research and Developments
Researchers around the world are actively working to improve the visual capabilities of multimodal LLMs. Advances in deep learning, computer vision, and data processing are paving the way for more sophisticated models that can better handle visual information.
Recent studies have shown promising results in enhancing the visual understanding capabilities of these models, but much work remains to be done.
Potential Solutions
Enhanced Training Data
One potential solution to the visual shortcomings of multimodal LLMs is the use of enhanced training data. By incorporating more diverse and high-quality visual data into the training process, models can learn to better recognize and interpret images.
Hybrid Models
Another approach is the development of hybrid models that combine the strengths of different AI architectures. These models can leverage the capabilities of both language and vision models to provide a more comprehensive understanding of multimodal data.
Challenges Ahead
While progress is being made, several challenges remain. These include the need for more robust evaluation metrics, the development of ethical guidelines for AI use, and the ongoing challenge of ensuring model transparency and explainability.
Conclusion and Future Directions
Multimodal LLMs hold immense promise for advancing artificial intelligence, but their visual shortcomings cannot be ignored. By addressing these limitations, developers can create more effective and reliable models that can be applied across a wide range of industries.
Future research should focus on enhancing the visual capabilities of these models through improved training data, advanced architectures, and interdisciplinary collaboration.
Call to Action
We invite you to engage with this topic further by leaving your thoughts in the comments section below. Share your insights on the visual shortcomings of multimodal LLMs and how you believe they can be addressed. Additionally, explore other articles on our site to deepen your understanding of AI and its applications.
Data sources and references for this article include reputable journals such as Nature Machine Intelligence, IEEE Transactions on Pattern Analysis and Machine Intelligence, and research papers from leading AI institutions.
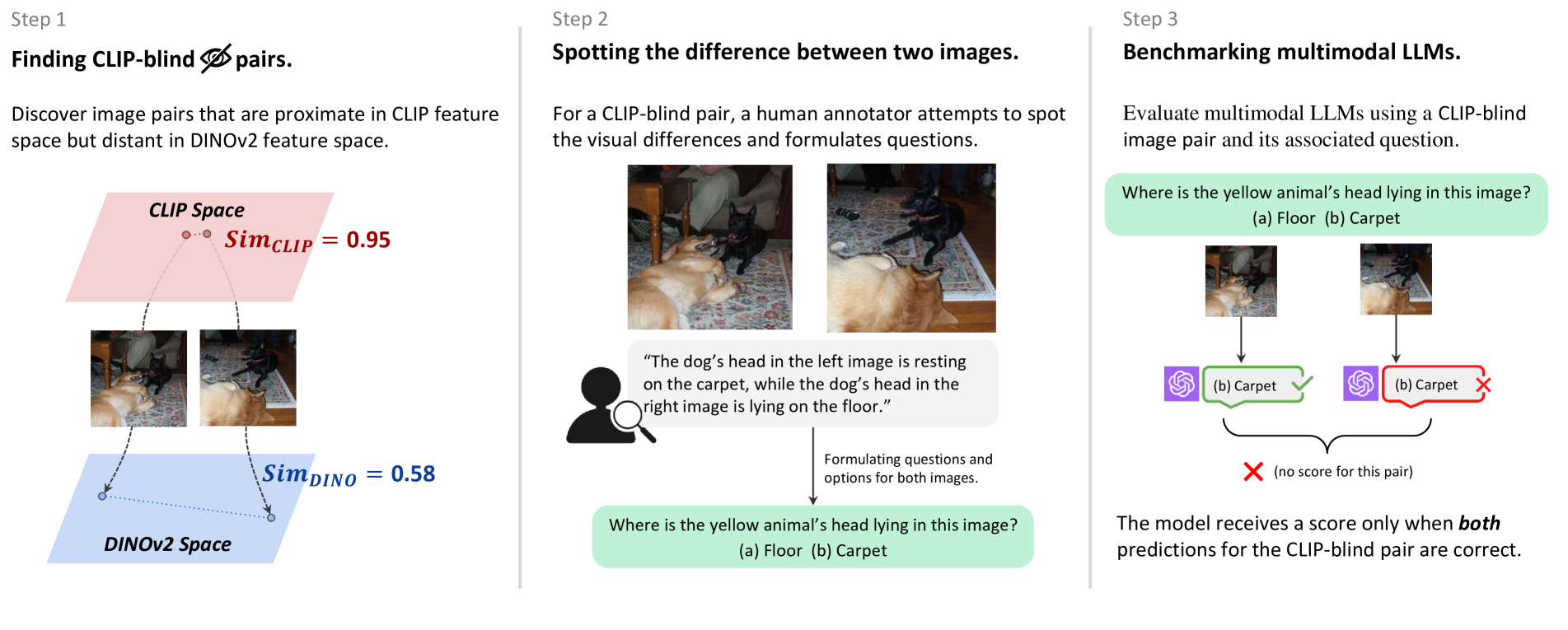